Predictive Analysis Techniques: Harnessing Data for Future Insights
Predictive analysis is a branch of data analytics aimed at making predictions about future outcomes based on historical data combined with statistical modeling, data mining techniques, and machine learning. As businesses and organizations generate ever-increasing volumes of data, the significance of predictive analytics is becoming more prominent in decision-making processes across various industries. This article explores key techniques and examples of predictive analysis, highlighting its benefits and applications.
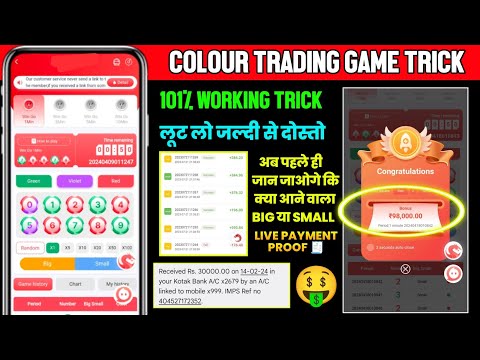
Key Techniques in Predictive Analysis
Predictive analysis encompasses a variety of statistical and machine learning techniques. Here are some of the most widely used:
- Regression Analysis: Used to understand relationships between variables and forecast numeric values. Linear and logistic regression are two common forms.
- Decision Trees: A model that uses a tree-like graph of decisions and their possible consequences, including chance event outcomes and resource costs.
- Neural Networks: Inspired by biological neural networks, these systems learn tasks by considering examples, generally without task-specific programming.
- Time Series Analysis: Involves analyzing time-ordered data points to extract meaningful statistics and identify characteristics of the data.
.png)
Applications of Predictive Analysis
Here are several examples of how predictive analysis is being deployed across different sectors:
- Finance: Credit scoring and risk assessment are primary applications of predictive analytics in finance, helping banks to decide who qualifies for a loan and at what interest rates.
- Healthcare: Predictive models in healthcare help foresee admission rates and possible outbreaks, improving service delivery in hospitals.
- Marketing: Predictive analytics help forecast customer responses or purchases, as well as promote cross-sell opportunities. Analyzing customer behavior patterns leads to more effective marketing strategies.
- Retail: Retailers use predictive models to manage inventory and personalize marketing efforts to individual customer preferences and buying habits.
- Manufacturing: Predictive maintenance techniques are used to anticipate equipment failures before they happen, reducing downtime and maintenance costs.
Benefits of Predictive Analysis
Implementing predictive analytics can provide substantial benefits to a business, including:
- Improved Decision Making: Provides actionable insights that drive smarter, data-driven decisions.
- Enhanced Efficiency: Optimizes processes and improves operational efficiencies by predicting the likelihood of various scenarios.
- Increased Revenue: Helps identify and maximize opportunities for up-selling and cross-selling.
- Risk Reduction: Assists in identifying potential risks and mitigating them through better strategic planning.
.png)
Conclusion
The potency of predictive analytics lies in its capability to not only interpret the past and assess the present but also to forecast the future with a reasonable degree of accuracy. By employing techniques such as regression analysis, decision trees, and neural networks, businesses can anticipate market trends, understand customer behavior, and make informed decisions that bolster growth and efficiency. As technology evolves, predictive analytics will become increasingly integral in shaping future strategies across all sectors.